Piet Seuntjens is program manager of digital water at VITO. He holds a master’s degree in bio-engineering, a PhD in sciences and is visiting professor at the University of Antwerp. He has track record in managing innovation programs related to modelling and monitoring of water systems.
In this blog, he further develops his understanding of digitalization in the water sector, aiming to make the transition to more informed, sometimes called smarter or intelligent water operations and management.
Digitalization is a broad term, covers many aspects, and is ill-defined on many occasions. To his opinion, digitalization concerns not purely the digital aspect, as it requires the combination of domain knowledge, conceptual and holistic thinking, modelling and ICT skills. This is reflected in the organization of VITO’s digital water program comprising domain knowledge on water, conceptual thinking, data analytics and predictive modelling. Close connection with ICT experts thinking along with the business is key to provide successful products such as models, webservices, decision support tools, and digital twins.
Digitalisation, a broad term
The ‘Fourth Industrial Revolution’, Industry 4.0 and ‘The Second Machine Age’ are used to describe current efforts to digitalize all kinds of sectors. Digitalization is also taking place in different fields of resource management: energy, waste and water. The digitalization of the water sector is a basis for holistic management of water networks (natural water, distribution networks), water treatment, water reuse and resource recovery. In the broader context of circular economy, digital technologies are an enabler for the upscaling as they allow to create and process data and information required for circular business models and the complex demands of circular supply chains.
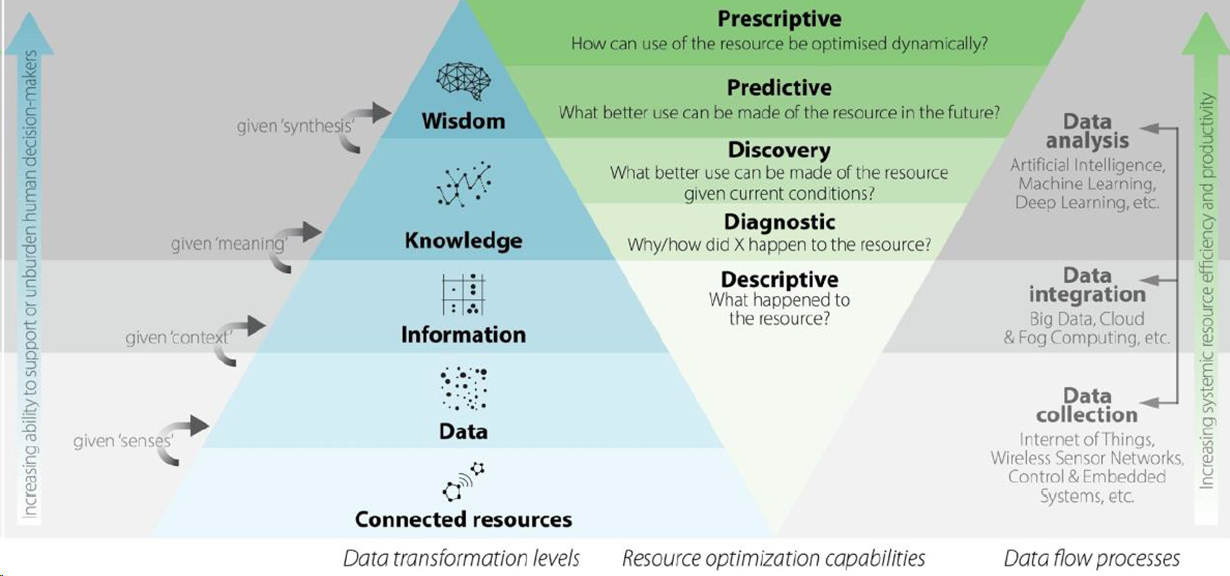
In the urban environment, digitalization is also at the very core of the development of smart cities where interconnected services such as mobility, environment, health, energy, water and circular economy are being developed as urban digital twins. This also calls for common understanding of terms and concepts, architectures, and standards allowing for interoperable services where sensors, things, data and models can be more easily connected (Lefever et al. 2019).
Why digital water?
The growing concerns about worldwide freshwater resources for human consumption impose increasing attention and focus on the monitoring, assessment, and protection of these resources. Indeed, information and communication technologies (ICT) are currently playing a key role in the observation of water systems, both for what are regarded as man-made infrastructures and for those that are regarded as being water in its natural form. Examples are new observational approaches, direct sensing techniques, sensor networking architectures, data processing and analysis methods, and integration with large data systems.
The role of data as a vital resource that enhances decision making and which supports efficient systems operation has become evident, with a growing number of water supply companies viewing data as a key organizational aspect that must be properly managed.
In 2015 and 2016, the EU has established a roadmap towards a Single Digital Market for water services, followed by an action plan document ICT4Water cluster (2019).
These documents spread a strong belief in the opportunities of connecting the digital world of metering and observation with advanced water treatment and distribution systems that can lead us to unimaginable levels of manageability and control within smart cities, factories of the future and the water-smart society at large.
This is also acknowledged by IWA, the world’s leading water sector organization in its report Digital Water, 2019 (IWA, Digital Water, 2019). Anticipated benefits of digitalization in the water sector and its transformation towards circular business are (Mbavarira and Grimm, 2021):
- Reducing energy consumption
- Avoiding unnecessary water losses
- Minimizing resource consumption
- Better monitoring on quality, quantity, reuse of water, extreme events, and informed decision making
- Engaging end consumers and awareness raising of water consumption patterns
Digital drinking water
Drinking water treatment plants are designed and operated to treat contaminants known to occur in raw water, comply with drinking water standards, and meet customer expectations.
Online monitoring of several common parameters of water quality is used in water distribution systems to ensure safety for drinking and sanitation. Unanticipated changes in quality or the presence of unusual contaminants in source water can adversely impact the ability of a utility to meet these objectives.
Watershed monitoring can improve a utility’s ability to detect variations in source water quality. Monitoring involves the measurement of various water quality parameters such as salinity, turbidity, pH, nitrate in source water or watersheds.
Monitoring locations are selected relative to control points, which are locations where a treatment process can be modified (e.g., addition of pretreatment chemicals) or a response action can be implemented (e.g., closing an intake).
The scale of a monitoring system can extend from an individual drinking water utility monitoring at its treatment plant intake to systems that monitor an entire watershed. The latter typically involve multiple organizations to provide coverage of a large area (e.g., an entire watershed or river basin) and share the cost required to install, operate, and maintain the system.
Benefits of a watershed-scale monitoring system include the ability to achieve extensive geographic coverage and maintain more monitoring locations than could be maintained by any single organization. However, such systems require sustained commitment by all partners and can present challenges if partner organizations decide to end their support. An example of a watershed monitoring system is the Internet of Water Flanders initiative (internetofwater.be).
In the subsequent blog, Maarten Van Loo will further develop on the forecasting capabilities of machine learning on river water quality data to forecast water quality at a drinking water intake.
Digital wastewater and water resource recovery
Wastewater or water resource recovery systems can vary in both size and complexity ranging from small and simple rural catchments to large and complex urban settings.
In the wastewater collection network, historically, there has been a lack of permanent wastewater monitoring because of the propensity for fouling and the complications of monitoring networks. The wastewater treatment works tend to be much better monitored depending upon the size of the works.
However, this monitoring has been very much based upon single system instrument-based control systems (e.g., a dissolved oxygen control system for an activated sludge plant).
The future of both the wastewater network and the wastewater treatment works will be a much more holistic approach bringing the network and the treatment works together and treating it as a single system, based upon the philosophy of the resource factory (water resource recovery facility or WRRF) and treating the outputs from the wastewater treatment works as a product.
In this way, rather than operating the wastewater treatment system for process control with the aim of protecting the water environment, it can also be operated for resource recovery and energy efficiency with a much wider environmental benefit. A notable example of an instrumented and controlled WRRFs are the Tilburg and Eindhoven plants of Waterschap De Dommel (wastewater.ai).